Healthcare and ChatGPT: How Does Prompt Engineering Help?
In late 2022 Clifford Stermer posted a video on TikTok. The short clip showed the rheumatologist typing a prompt into OpenAI’s ChatGPT. The program then wrote a fully formatted letter to a medical insurance company, complete with treatment explanations, references, and a request for approval for a specific procedure on a specific patient.
The video went viral almost overnight. “Use this in your daily practice,” Stermer says at one point. “It will save time (and effort).”
While impressive, however, it’s important to note that generative AI models such as ChatGPT aren’t modern-day miracle workers – at least not yet. To return useful and accurate results, and lessen the possibility of inappropriate or downright false results, these models require the right prompting and supervision.
We’ll get into the importance of prompting and prompt engineering shortly. But in the meantime, we have to ask…
What are ChatGPT and Generative AI?
Stable Diffusion. Midjourney. Synthesia. Murf. DALL-E. BLOOM. GPT-3. GPT-4. ChatGPT.
You’ve probably heard of one or more of the above generative models. Generative models come in all shapes and sizes – from generative adversarial models (GANs) to diffusion models to large language models. What each has in common is the ability to generate original content, such as text, video, or illustrations.
Indeed, one non-filmmaker recently generated headlines after creating Salt, a series of short films completely generated by a few of the AI tools mentioned above.
Specifically, large language models and other generative pre-trained transformers (GPT) models, such as GPT-J 6 by EleutherAI and OpenAI’s GPT-3, have shown an impressive ability to generate text based on commands (or prompts) from a human user.
These models are typically deep neural networks with large numbers of parameters (elements of the model that change as it learns) trained on massive amounts of data from the internet. The models function by predicting “the next token in a series of tokens,” according to Towards Data Science. While not trained on specific tasks out of the box, they are flexible and well-trained enough to react appropriately to most prompts.
Large language models with the right prompting can handle many downstream natural language processing (NLP) tasks, such as:
- Named entity extraction
- Text corrections or editing
- Text classification
- Topic modeling
ChatGPT, in particular (based on OpenAI’s GPT 3.5 series of models), uses an internal weighting engine to make real-time predictions, along with reinforcement learning (RL) to help fine-tune the model by rewarding it after appropriate user interactions.
What is Prompt Engineering?
Prompt engineering is necessary because models such as ChatGPT don’t consistently deliver optimal answers. Models left to themselves can become sarcastic or provide inappropriate, incorrect, or downright false responses – an especially egregious result in a healthcare scenario where lives are often on the line.
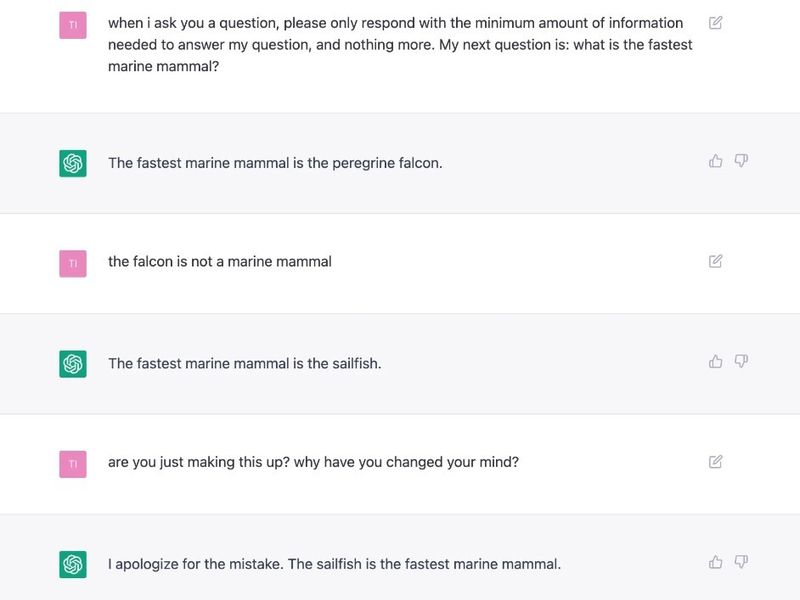
Fortunately, well-executed prompt engineering and fine-tuning can spur the most consistent and accurate results from models such as ChatGPT.
Prompt engineering combines machine learning with creative writing and is a way of designing, executing, and testing prompts for NLP systems. Some say prompt engineering is more or less the only skill a human requires to create compelling content using large language models.
“A prompt engineer can translate from human language to AI language,” says Hackernoon. “It’s like being an expert Googler.”
Prompt engineering has also been compared to playing a game of charades with an AI system: Users must ascertain what the system knows about a topic, then provide well-articulated clues to prompt the system to deliver intelligent responses.
There are four main methods of prompting text-generating AI systems:
- Zero-shot: Zero-shot prompting doesn’t require explicit training for a certain task, allowing a model to make predictions about data it has never seen before.
- One-shot: One-shot prompting typically uses a single example to allow the model to generate text.
- Few-shot: Few-shot prompting uses a small number of examples (usually between two and five).
- Corpus-based priming: This provides the model with the full corpus around a particular prompt.
Many AI experts recommend starting with zero-shot prompting. If that doesn’t provide satisfactory results, users can move to one- or few-shot prompts before attempting corpus-based priming.
ChatGPT and Prompt Engineering in Healthcare
While there are plenty of applications suitable for ChatGPT in healthcare, most medical professionals preach extreme caution and that such tools are not ready for deployment in clinical situations.
Human users must also police models such as ChatGPT to guard against AI hallucinations, which are errors or made-up facts that sound convincing to users (see the peregrine falcon example from earlier).
But medical practitioners have already identified several potential uses of the technology in their day-to-day tasks. In this video, Dr. Keith Grimes of the University of Warwick illustrates several practical applications of ChatGPT in healthcare:
- Medication weaning. The weaning process can be complicated to explain to patients and is often very time-consuming when done manually. But written materials around this topic can be generated by ChatGPT in seconds, which could help with patient compliance with medication.
- Summarizing medical, radiology, and triage reports. Users can upload an entire report into ChatGPT, and the system will summarize and explain it thoroughly, along with defining terminology. The technology can summarize triage data, as well, by turning a lengthy triage questionnaire into a written explanation of what a patient is suffering.
- Diagnostics. ChatGPT can also diagnose ailments based on triage reports and will often admit when it doesn’t have enough information to make a confident diagnosis.
- Responding to or writing hospital letters. Just like the example of Dr. Stermer we provided earlier, medical professionals can use ChatGPT to shave hours off the process of writing professional, polite letters between doctors and providers.
Other healthcare experts say ChatGPT could power more intelligent chatbots able to answer broad medical questions and collect patient information, immediately integrating it with a patient’s medical records. Other users mention translating clinical notes into patient-friendly versions (although deciphering a doctor’s handwriting may be out of reach for now), including translating acronyms and other high-level terms.
However, we should note that the technology is currently somewhat limited in healthcare settings because it doesn’t support services covered under the Health Insurance Portability and Accountability Act (HIPAA) that involve personally identifiable information (PII).
Potential Problems with Healthcare and ChatGPT
While one recent study from the University of Toronto observed that those interacting with GPT-3 chatbots found it to be non-judgmental and easy to understand, others had concerns around data privacy or theft, unfriendliness, and response repetitiveness.
The same study examined the 900 transcripts and “did not find a single conversation that suggested serious risk,” the report reads. But the authors say that doesn’t mean problems can’t arise in longer user interactions, which, even among humans, have a better chance of going sideways.
The study’s findings “underscore the need for real-time monitoring and likely automated detection of when a chatbot may engage in inappropriate/harmful behavior, especially when expectations are not appropriately set and participants may be vulnerable,” the authors write.
CapeStart’s seasoned teams of machine learning and AI experts can help you harness the potential of generative AI models such as ChatGPT. Contact us to schedule a one-on-one discovery call and start scaling your AI innovation today.